Note
Go to the end to download the full example code.
1. Theta oscillation cycle feature distributions¶
Compute and compare the distributions of bycycle features for two recordings.
import numpy as np
import matplotlib.pyplot as plt
from neurodsp.filt import filter_signal
from neurodsp.plts import plot_time_series
from bycycle import BycycleGroup
from bycycle.plts.features import plot_feature_hist
from bycycle.utils.download import load_bycycle_data
Load and preprocess data¶
# Load data
ca1_raw = load_bycycle_data('ca1.npy', folder='data')
ec3_raw = load_bycycle_data('ec3.npy', folder='data')
fs = 1250
f_theta = (4, 10)
# Apply a lowpass filter at 25Hz
fc = 25
filter_seconds = .5
ca1 = filter_signal(ca1_raw, fs, 'lowpass', fc, n_seconds=filter_seconds,
remove_edges=False)
ec3 = filter_signal(ec3_raw, fs, 'lowpass', fc, n_seconds=filter_seconds,
remove_edges=False)
Compute cycle-by-cycle features¶
# Set parameters for defining oscillatory bursts
thresholds = {
'amp_fraction_threshold': 0,
'amp_consistency_threshold': .6,
'period_consistency_threshold': .75,
'monotonicity_threshold': .8,
'min_n_cycles': 3
}
# Cycle-by-cycle analysis
sigs = np.array([ca1, ec3])
bg = BycycleGroup(thresholds=thresholds, center_extrema='trough', return_samples=False)
bg.fit(sigs, fs, f_theta)
df_ca1, df_ec3 = bg.df_features
# Limit analysis only to oscillatory bursts
df_ca1_cycles = df_ca1[df_ca1['is_burst']]
df_ec3_cycles = df_ec3[df_ec3['is_burst']]
Plot time series for each recording¶
# Choose samples to plot
samplims = (10000, 12000)
ca1_plt = ca1_raw[samplims[0]:samplims[1]]
ec3_plt = ec3_raw[samplims[0]:samplims[1]]
times = np.arange(0, len(ca1_plt)/fs, 1/fs)
fig, axes = plt.subplots(figsize=(15, 6), nrows=2)
plot_time_series(times, ca1_plt, ax=axes[0], xlim=(0, 1.6), ylim=(-2.4, 2.4),
xlabel="Time (s)", ylabel="CA1 Voltage (mV)")
plot_time_series(times, ec3_plt, ax=axes[1], colors='r', xlim=(0, 1.6),
ylim=(-2.4, 2.4), xlabel="Time (s)", ylabel="EC3 Voltage (mV)")
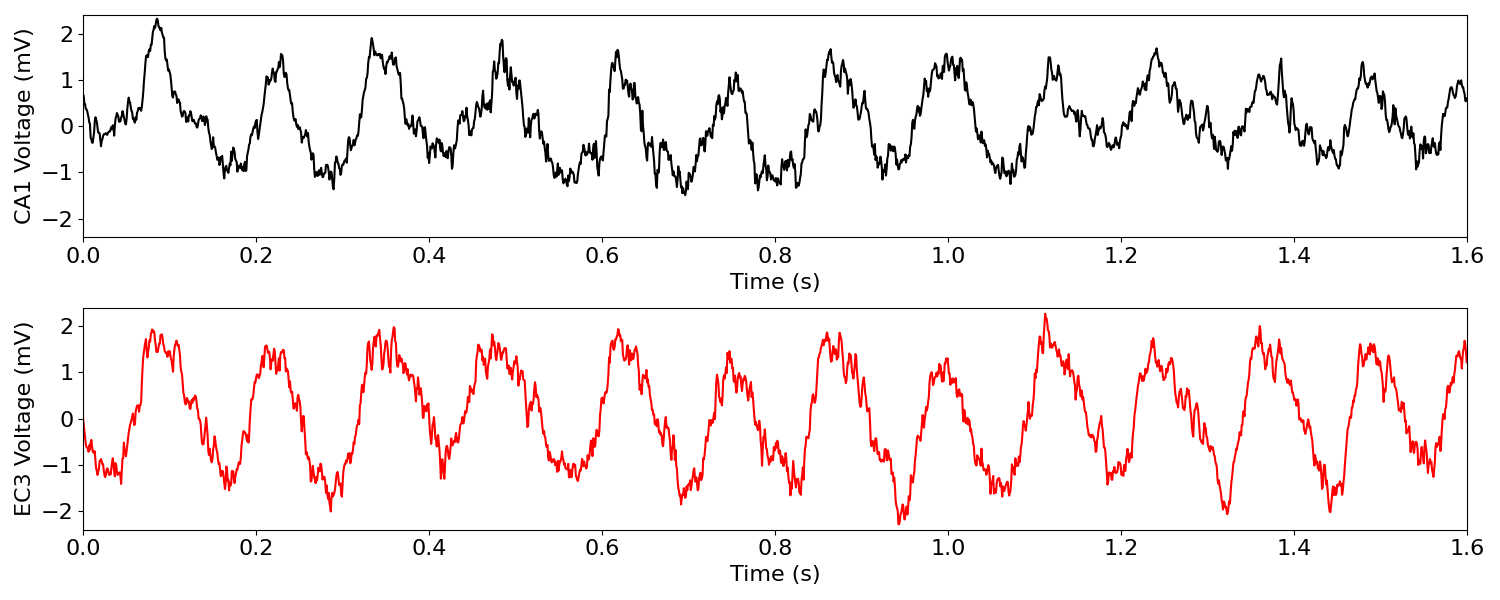
Plot feature distributions¶
fig, axes = plt.subplots(figsize=(15, 15), nrows=2, ncols=2)
# Plot cycle amplitude
cycles_ca1 = df_ca1_cycles['volt_amp']
cycles_ec3 = df_ec3_cycles['volt_amp']
plot_feature_hist(cycles_ca1, 'volt_amp', ax=axes[0][0], xlabel='Cycle amplitude (mV)',
xlim=(0, 4.5), color='k', bins=np.arange(0, 8, .1))
plot_feature_hist(cycles_ec3, 'volt_amp', ax=axes[0][0], xlabel='Cycle amplitude (mV)',
xlim=(0, 4.5), color='r', bins=np.arange(0, 8, .1))
axes[0][0].legend(['CA1', 'EC3'], fontsize=15)
# Plot cycle period
periods_ca1 = df_ca1_cycles['period'] / fs * 1000
periods_ec3 = df_ec3_cycles['period'] / fs * 1000
plot_feature_hist(periods_ca1, 'period', ax=axes[0][1], xlabel='Cycle period (ms)',
xlim=(0, 250), color='k', bins=np.arange(0, 250, 5))
plot_feature_hist(periods_ec3, 'volt_amp', ax=axes[0][1], xlabel='Cycle period (ms)',
xlim=(0, 250), color='r', bins=np.arange(0, 250, 5))
# Plot rise/decay symmetry
plot_feature_hist(df_ca1_cycles, 'time_rdsym', ax=axes[1][0], xlim=(0, 1), color='k',
xlabel='Rise-decay asymmetry\n(fraction of cycle in rise period)',
bins=np.arange(0, 1, .02))
plot_feature_hist(df_ec3_cycles, 'time_rdsym', ax=axes[1][0], xlim=(0, 1), color='r',
xlabel='Rise-decay asymmetry\n(fraction of cycle in rise period)',
bins=np.arange(0, 1, .02))
# Plot peak/trough symmetry
plot_feature_hist(df_ca1_cycles, 'time_ptsym', ax=axes[1][1], color='k',
xlabel='Peak-trough asymmetry\n(fraction of cycle in peak period)',
bins=np.arange(0, 1, .02))
plot_feature_hist(df_ec3_cycles, 'time_ptsym', ax=axes[1][1], color='r',
xlabel='Peak-trough asymmetry\n(fraction of cycle in peak period)',
bins=np.arange(0, 1, .02))
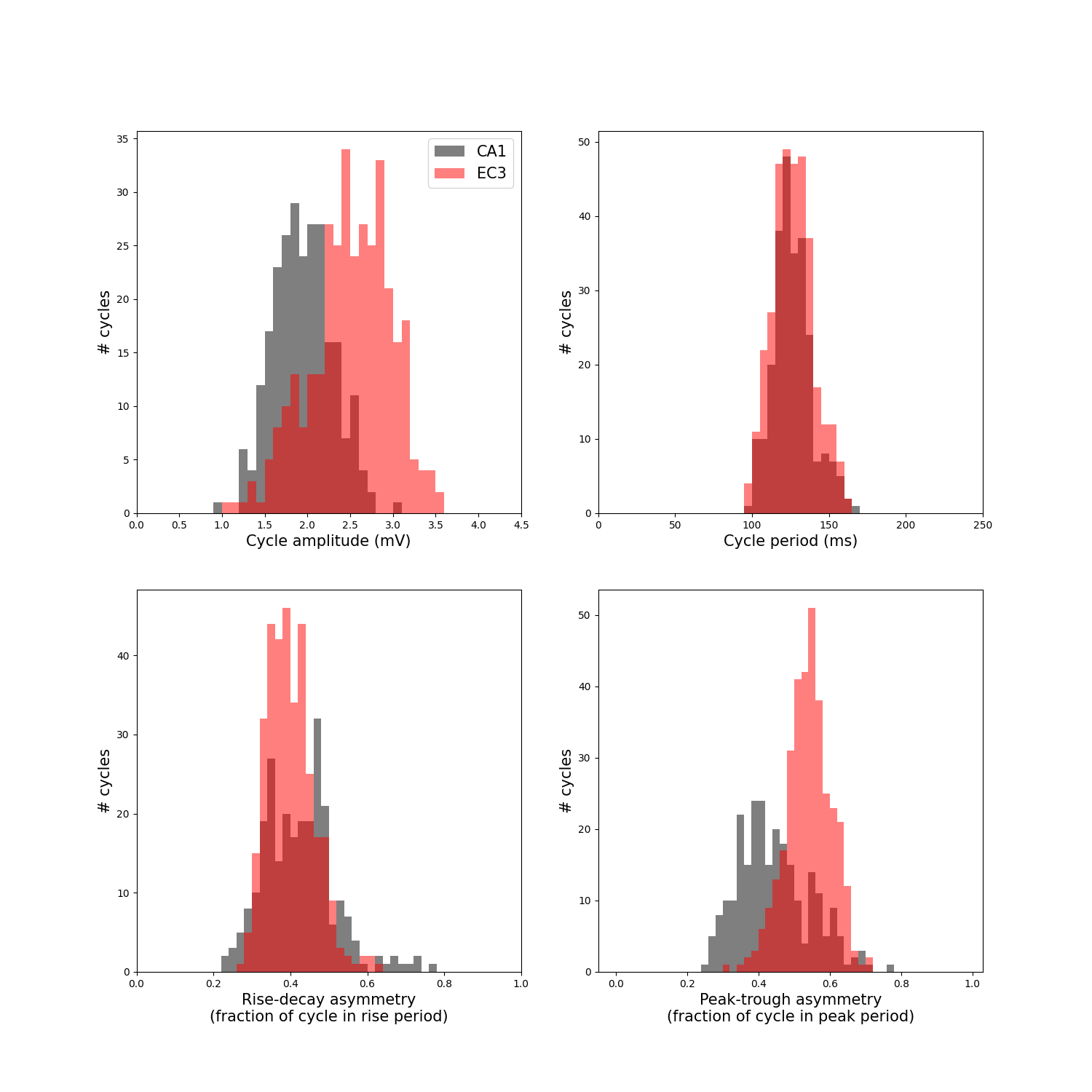
Total running time of the script: (0 minutes 1.045 seconds)